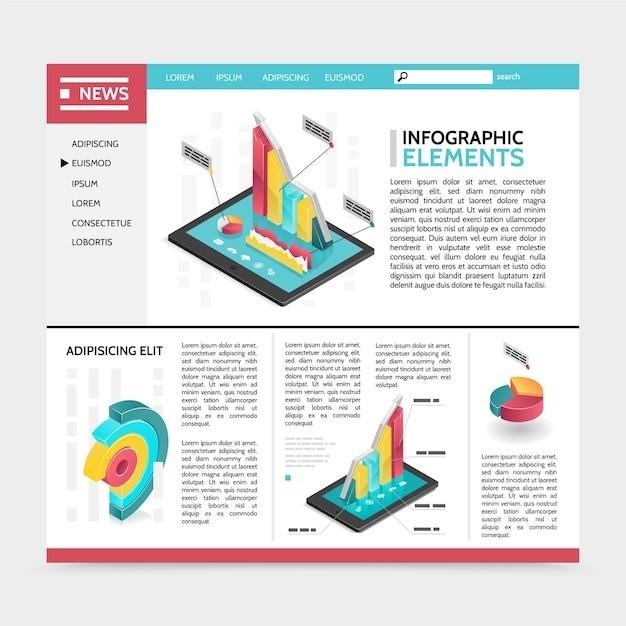
Applied Statistics for Business and Economics⁚ A Comprehensive Overview
Applied statistics is a powerful tool for businesses and economists, enabling them to analyze data, make informed decisions, and understand complex trends. This comprehensive overview explores the fundamental concepts of applied statistics, its practical applications, and essential software tools used in various fields, including business, finance, and economics.
Introduction⁚ The Importance of Statistics in Business and Economics
In today’s data-driven world, statistics has become an indispensable tool for businesses and economists alike. The ability to analyze data, draw meaningful insights, and make informed decisions based on evidence is crucial for success in these fields. Applied statistics provides a framework for understanding and interpreting data, enabling organizations to make better decisions, optimize processes, and gain a competitive edge.
For businesses, statistics plays a vital role in various aspects, including market research, customer segmentation, sales forecasting, inventory management, and quality control. By analyzing customer data, businesses can identify patterns, understand customer preferences, and tailor their marketing strategies for maximum effectiveness. Similarly, economists use statistical methods to analyze economic indicators, predict future trends, and develop sound economic policies.
From understanding consumer behavior to evaluating the effectiveness of marketing campaigns, statistics empowers businesses to make data-driven decisions that lead to improved profitability and sustainable growth. In the realm of economics, statistics helps to quantify economic phenomena, measure inflation, and assess the impact of government policies on the economy. Through statistical analysis, economists can identify key drivers of economic growth, understand the causes of recessions, and develop models to forecast future economic performance.
Understanding the Fundamentals of Applied Statistics
At the heart of applied statistics lies a set of fundamental concepts that form the foundation for data analysis and interpretation. These concepts provide the tools and frameworks necessary to extract meaningful insights from data and make informed decisions. A thorough understanding of these fundamentals is essential for anyone seeking to apply statistics in business or economics.
One key concept is the distinction between descriptive and inferential statistics. Descriptive statistics focuses on summarizing and presenting data in a meaningful way, using measures like mean, median, mode, standard deviation, and variance. This allows for a clear understanding of the data’s central tendency, dispersion, and distribution. Inferential statistics, on the other hand, goes beyond simply describing data and aims to draw conclusions about a population based on a sample of data. This involves using statistical tests to assess hypotheses and make predictions about future outcomes.
Another fundamental concept is the idea of probability and probability distributions. Probability deals with the likelihood of events occurring and provides a framework for quantifying uncertainty. Probability distributions, such as the normal distribution, binomial distribution, and Poisson distribution, describe the probability of different outcomes in a given scenario. Understanding probability and probability distributions is crucial for making informed decisions under uncertainty, a common challenge in business and economics.
Data Collection and Preparation
The foundation of any statistical analysis lies in the quality and integrity of the data collected. Before embarking on any statistical analysis, it is crucial to ensure that the data is accurate, relevant, and properly prepared for analysis. Data collection and preparation are often overlooked but are critical steps that can significantly impact the reliability and validity of the results.
Data collection involves gathering relevant information from various sources. These sources can include surveys, experiments, databases, historical records, or even publicly available datasets. It’s important to choose appropriate methods for data collection based on the specific research question and the nature of the data being sought. For example, surveys are suitable for gathering opinions and preferences, while experiments are ideal for testing causal relationships.
Once data is collected, it needs to be carefully prepared for analysis. This preparation process involves cleaning the data to remove errors, inconsistencies, or missing values. It also includes transforming the data into a format suitable for statistical analysis, such as organizing it into tables, creating new variables, or converting data types. This meticulous preparation ensures that the data is ready for meaningful analysis and that the results are reliable and accurate.
Descriptive Statistics⁚ Summarizing Data
Descriptive statistics provides a powerful toolkit for summarizing and understanding large datasets. It allows us to condense vast amounts of information into meaningful measures that reveal the key characteristics of the data. These measures help us gain insights into the central tendency, spread, and distribution of the data, providing a foundation for further analysis.
Measures of central tendency, such as the mean, median, and mode, provide a single value that represents the typical or average value of the data. The mean represents the average value, the median represents the middle value when data is ordered, and the mode represents the most frequent value. These measures help us understand the center of the data distribution.
Measures of spread, such as the range, variance, and standard deviation, quantify the variability or dispersion of the data points around the central tendency. The range represents the difference between the highest and lowest values, while variance and standard deviation measure how spread out the data is from the mean. These measures help us understand the heterogeneity of the data.
Probability and Probability Distributions
Probability is the foundation of statistical inference, providing a framework for quantifying uncertainty and making predictions based on data. It allows us to calculate the likelihood of events occurring, which is crucial in decision-making under uncertainty. Probability distributions describe the probabilities of different outcomes in a random experiment, providing a comprehensive picture of the possible results.
Common probability distributions include the normal distribution, binomial distribution, and Poisson distribution. The normal distribution, often referred to as the bell curve, is a symmetrical distribution used to model continuous variables, such as heights or weights. The binomial distribution models the probability of success in a fixed number of trials, such as the number of heads in coin flips. The Poisson distribution models the number of events occurring in a fixed interval of time or space, such as the number of customers arriving at a store in an hour.
Understanding probability and probability distributions allows us to assess the likelihood of events, make informed decisions based on uncertain information, and quantify the risks associated with different choices. This knowledge is crucial in various applications, including forecasting, risk management, and investment decisions.
Statistical Inference⁚ Drawing Conclusions from Data
Statistical inference is the process of using sample data to draw conclusions about a larger population. It allows us to make generalizations and predictions about the characteristics of a population based on the information gathered from a subset of individuals. This process involves two main branches⁚ estimation and hypothesis testing.
Estimation involves using sample statistics to estimate population parameters. For example, we might use the average income of a sample of employees to estimate the average income of all employees in a company. Hypothesis testing involves formulating hypotheses about population parameters and using sample data to determine whether there is enough evidence to reject or support these hypotheses. This allows us to test claims and make informed decisions based on data.
Statistical inference is essential for businesses and economists to make informed decisions, analyze trends, and assess the effectiveness of various strategies. By drawing conclusions from data, we can gain valuable insights into the population, identify patterns, and make predictions about future outcomes.
Hypothesis Testing⁚ Evaluating Claims
Hypothesis testing is a crucial aspect of applied statistics, allowing us to evaluate claims and make informed decisions based on data. It involves formulating a null hypothesis, which represents the status quo or a statement we want to disprove, and an alternative hypothesis, which is the claim we want to support. The process involves collecting data, calculating a test statistic, and comparing it to a critical value or p-value to determine whether there is enough evidence to reject the null hypothesis.
For example, a business might want to test the hypothesis that a new marketing campaign will increase sales. The null hypothesis would be that the campaign has no effect on sales, while the alternative hypothesis would be that it increases sales. By analyzing sales data before and after the campaign, they can determine whether there is enough evidence to reject the null hypothesis and support the claim that the campaign was successful.
Hypothesis testing is widely used in business and economics to assess the effectiveness of marketing strategies, analyze the impact of economic policies, and determine the significance of trends and relationships. By providing a framework for evaluating claims, hypothesis testing helps us make informed decisions and draw meaningful conclusions from data.
Regression Analysis⁚ Predicting Relationships
Regression analysis is a powerful statistical technique used to model and predict the relationship between variables. It allows us to understand how changes in one variable affect another, enabling us to make informed predictions and decisions. In business and economics, regression analysis is widely applied to forecast sales, estimate costs, analyze the impact of marketing campaigns, and assess the relationship between economic indicators.
For example, a company might use regression analysis to predict future sales based on historical data and other relevant factors like advertising spending, seasonal trends, and economic conditions. This helps them make strategic decisions regarding inventory management, pricing, and marketing investments. Similarly, economists might use regression analysis to study the relationship between interest rates and inflation, helping them understand the impact of monetary policy on economic growth.
The results of regression analysis are often presented in the form of a regression equation, which provides a mathematical representation of the relationship between variables. This equation allows us to predict the value of one variable based on the values of other variables, providing valuable insights for decision-making in business and economic contexts.
Time Series Analysis⁚ Forecasting Future Trends
Time series analysis is a specialized branch of statistics focused on analyzing data collected over time. This method helps us understand patterns, trends, and seasonal variations within data, enabling businesses and economists to make accurate forecasts about future outcomes. By examining historical data, we can identify recurring trends, seasonal fluctuations, and irregular events that may influence future performance.
For businesses, time series analysis can be used to forecast sales, demand, inventory levels, and other important metrics. This allows them to optimize production, manage inventory effectively, and make informed decisions about pricing and marketing strategies. Economists use time series analysis to predict economic growth, inflation, unemployment rates, and other key indicators, providing valuable insights for policymakers and investors.
The techniques used in time series analysis include moving averages, exponential smoothing, and autoregressive integrated moving average (ARIMA) models. These methods help us identify and quantify patterns in time series data, allowing us to make predictions about future values. Time series analysis is essential for businesses and economists who need to understand past trends and make informed decisions about the future.
Applications of Statistics in Business and Economics
Applied statistics plays a crucial role in both business and economics, providing valuable insights and supporting data-driven decision-making. In business, statistics is used to analyze market trends, understand consumer behavior, optimize pricing strategies, and forecast sales. For example, businesses can use statistical methods to analyze customer data and identify patterns in purchasing behavior, allowing them to tailor marketing campaigns and product offerings to specific customer segments.
In economics, statistics is essential for understanding economic indicators, forecasting economic growth, and evaluating the effectiveness of government policies. Economists use statistical models to analyze data on inflation, unemployment, GDP, and other key economic variables. These analyses help policymakers understand the state of the economy, identify potential problems, and develop effective policies to address them.
Statistical methods are also used in financial analysis to assess risk, manage investments, and make informed financial decisions. For example, statistical models can be used to evaluate the performance of different investment strategies, assess the risk of loan defaults, and predict future market trends. The applications of statistics are vast and continue to grow as data collection and analysis become increasingly sophisticated.
Software Tools for Applied Statistics
A wide array of software tools are available to assist in the application of statistical methods in business and economics. These tools streamline data analysis, provide advanced statistical functions, and facilitate the creation of visualizations for effective communication of findings. Some of the most widely used software packages for applied statistics include⁚
SPSS (Statistical Package for the Social Sciences)⁚ This user-friendly software is popular for its intuitive interface, extensive statistical functions, and capabilities for data management, analysis, and visualization. It’s widely used in social sciences, business, and healthcare.
R⁚ A free and open-source programming language and environment, R is favored for its extensive statistical capabilities and flexibility. It offers a wide range of packages for various statistical analyses, making it a powerful tool for research and development.
SAS (Statistical Analysis System)⁚ A comprehensive software suite known for its advanced statistical capabilities, data management, and reporting features. It’s commonly used in large organizations for data analysis, business intelligence, and decision support.
Stata⁚ A statistical software package known for its efficiency and user-friendly interface. It offers a wide range of statistical functions, data management tools, and graphical capabilities, making it suitable for research and analysis in various fields.
The Power of Applied Statistics in Decision-Making
In the realm of business and economics, applied statistics serves as a powerful tool for informed decision-making. By harnessing the power of data analysis, businesses can identify trends, predict future outcomes, and make strategic choices that drive growth and profitability. Applied statistics empowers economists to understand complex economic phenomena, model economic relationships, and contribute to sound economic policies.
Through the use of statistical methods, businesses can⁚
- Optimize operations⁚ Identify inefficiencies, improve processes, and reduce costs.
- Target marketing campaigns⁚ Understand customer behavior, segment markets, and tailor marketing efforts for greater effectiveness.
- Forecast sales and demand⁚ Improve inventory management, minimize stockouts, and maximize revenue.
- Assess risk and uncertainty⁚ Make informed decisions about investments, financial planning, and risk mitigation.
The application of statistical principles provides businesses and economists with a framework for data-driven decision-making, enabling them to navigate the complexities of the modern world with greater confidence and accuracy.